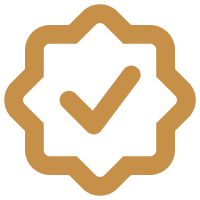
More precise detection of wafer/chip defects
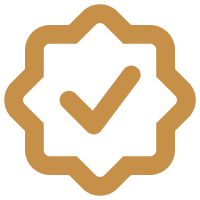
Automated classification of defect types
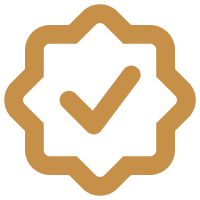
Stronger pattern defect detection capabilities
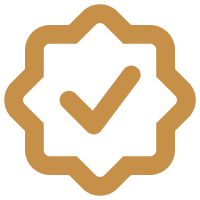
Significantly improved detection efficiency
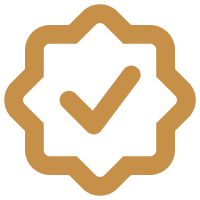
Reduced human intervention and subjective errors
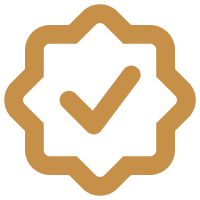
Enhancing defect detection for light guide plates and diffusion boards
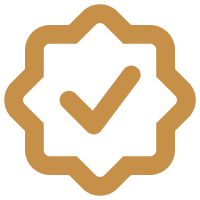
Accurate detection of fine circuit pattern defects
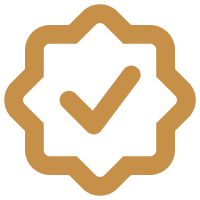
Improved detection rate for color filter and CF defects
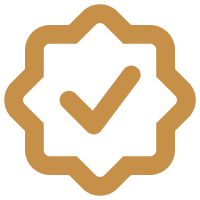
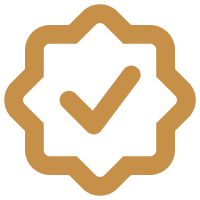
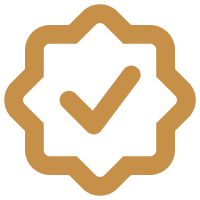
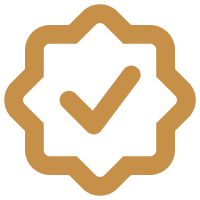
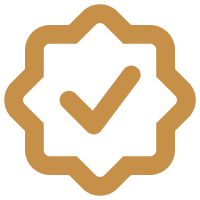
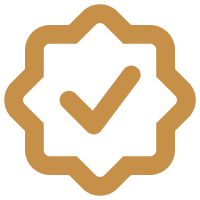
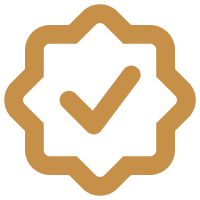
Enhancing defect detection for complex metal/mechanical components
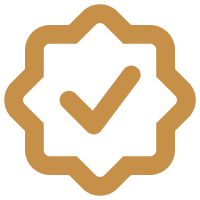
Handling diverse metal materials and surface characteristics
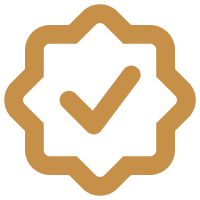
Improving defect detection for critical functional components
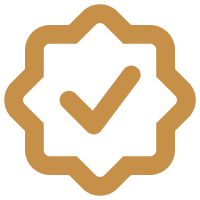
Shortening programming time for complex product inspections
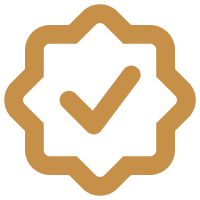
Enhancing high-speed production line inspection adaptability
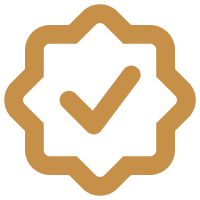
Real-time problem identification and resolution
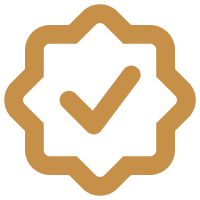
Reducing human errors
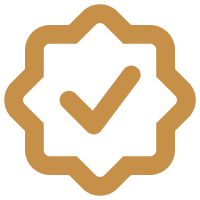
Reducing customer complaints and increasing ROI
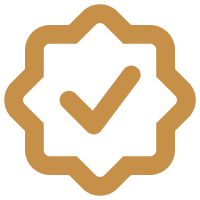
Lowering production costs
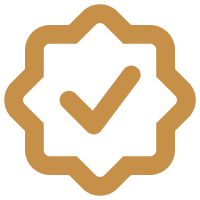
More precise detection of food surface defects
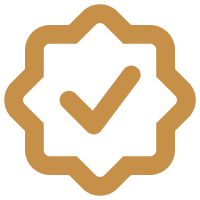
Accurate identification of foreign objects within food
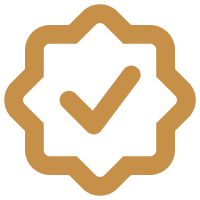
Automated food shape recognition and grading
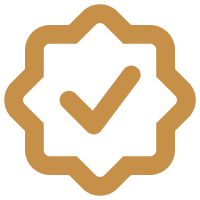
Rapid inspection of food packaging integrity
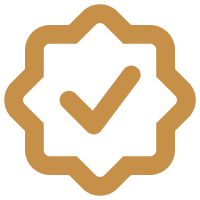
Improved detection of subtle surface defects on medical devices
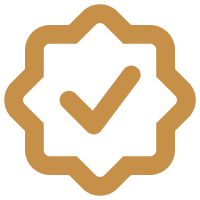
Accurate inspection of medical packaging integrity
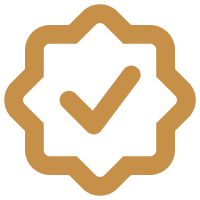
Realization of medical label character recognition
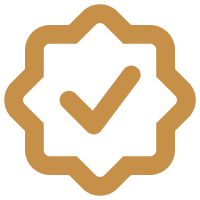
Improved inspection efficiency and reduced human risks
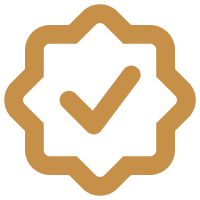
Improved detection accuracy
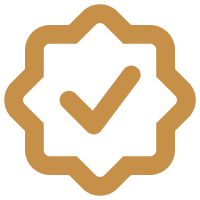
Flexible defect definition and classification
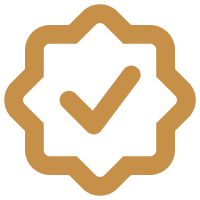
Highly adaptive optimization of inspection
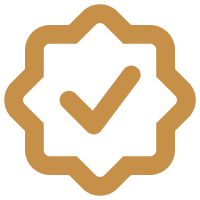
Automated, unattended operation
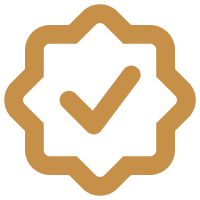
Improved detection of small defects on printed products
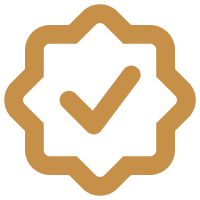
Accurate text/code recognition Labels/printed products often feature text, barcodes, and other encoded information.
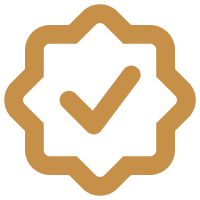
Efficient inspection of complex patterns and image defects
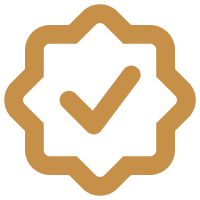
Significantly improved inspection efficiency
Semiconductor
Solutions:
Apply the Classification and Segmentation technology of Solomon SolVision AI image platform to identify defect features. First, use the Classification tool to judge whether the wafer has too many defects and eliminate the defective products that cannot be repaired. Then, use image processing technology to segment the wafer image, and use the Segmentation tool to detect the defects in the image, record their features, coordinates, area and other information, which greatly improves the efficiency of subsequent repairs.
- Web-based architecture, allowing multiple users to log in remotely through the domain
- Integrate and store a large amount of defect data and images detected by AOI equipment, which can be used for production history statistical analysis, real-time monitoring of online AOI equipment defect detection status, defect photo viewing, product defect Map overlay and defect type judgment Code and other functions
- Can be combined with AI for big data analysis and feedback to production equipment to issue warnings for production anomalies
Using the Segmentation technology of Solomon SolVision AI image platform, the image features of micro-scratches and dirt on the wafer are located and annotated, and then used to train the AI model. Even under the image background with grinding marks, AI vision can still easily detect deep and shallow micro-scratches and other dirt defects, and accurately detect the location and area of the defects.
- Line Scan high-speed detection + AI defect classification
- Support for front and back side appearance defect detection of Silicon/Glass Wafer
- Applicable to CIS/IQC/OQC
- Can be equipped with “8/12” EFEM, supports SECS GEM200/300
- High-speed automatic defect photography based on KLARF file for分区/分Die/Defect size/Defect type
- Provides AI real-time defect detection; instant detection and classification, processing speed up to 50 FPS or more
- Can be equipped with “8/12” EFEM, supports SECS GEM200/300
- Defect photography and detection of wafer/packaging process QA inspection stations/CP & FT
- AI real-time defect detection and classification, real-time detection and classification, processing speed can reach more than 50 FPS
- Can be equipped with “8/12” EFEM, supports SECS GEM200/300
- Min defect size ≧ 0.3µm
- Can be equipped with a yield management system
- High-speed inspection with a maximum inspection capacity of 60PCS/second (2M).
- AI deep learning identifies defects.
- Simultaneous inspection quantity can detect up to 10 areas at the same time.
- Inspection area 2mm2-200mm2.
- The inspection camera uses a 2M-25M high-speed area camera.
- The inspection accuracy camera is equipped with a lens resolution of 0.001mm-0.05mm.
- Measurement capability can detect 2M 60 frames/second or 5M 4 frames/second per second.
- Detailed measurement data classification is stored in different directory folders according to camera sequence, by date and order. Automatically create file names based on date and time.
- NG photo processing automatically generates directory folders and automatically creates file names based on date and time. During the inspection, click on the NG thumbnail or tile in the screen, and the complete photo can be popped up.
- Real-time measurement trend chart can display the data of the last 1000 points of each axis.
- The operation mode has operation mode (only display data), engineering mode (including real-time images) and commissioning mode.
- Cognex's Deep Learning tools apply intelligent algorithms to learn the difference between normal structural layers and defects, enabling more effective detection of tiny cracks.
- Highly accurate detection can save good chip packages that may have been incorrectly classified as defective (NG), thereby increasing yield. Deep learning can find tiny cracks on WLCSP packages that may pass traditional inspection methods but fail prematurely in the field.
Cognex Deep Learning software can perform automated defect screening for a larger portion of the wafer. The defect detection tool can completely ignore the underlying wafer layer, even finding small defects anywhere in the wafer layer, and then remove any outliers. It can also be used in a two-tier inspection system to identify ambiguous cases and send them to an offline manual inspection station for further review.
- Customized inspection requirements for different processes
- Precise quality judgment and classification of inspection results
- Inspection of product defect distribution map and individual defect features
- High-speed, non-contact, 3D/2D surface morphology measurement
Cognex's Deep Learning defect detection tools can find unacceptable and varied coating defects on wafer surfaces, which would be too complex or time-consuming for rule-based machine vision systems. The tool inspects the wafer surface, detecting if any cracks, chips or stains are present across it. It is trained on many different images showing variations in defect types and locations to identify potential areas of interest for inspection. Cognex's Deep Learning classification tools then classify the defects (e.g. cracks, chips, particles, etc.). This information can be used to improve processes to reduce defects and increase yield.
Apply the Segmentation technology of Solomon SolVision's AI image platform to build an AI learning module to automatically learn and detect the characteristics and location of crawling glue and overflowing glue. Combined with data augmentation technology, simulate the possible situations of adhesive overflow, so that AI can learn more feature patterns to improve accuracy. On the other hand, increasing the number of correct categories can improve the recognition strength and effectively reduce the interference of environmental factors.
Use SolVision's Feature Detection feature to learn the location points that need to be identified on the tray, and then use Segmentation technology to perform optical character recognition (OCR), which can greatly optimize the traditional AOI workflow. It is not restricted by the displacement, skew and character defects of the identification screen, and can accurately identify the source of individual materials. With the increase of the number of learning pieces, the ability of AI to identify characters can also be continuously optimized, making character identification no longer difficult.
Using SolVision AI image platform's unsupervised learning tool Anomaly Detection, AI deep learning is performed with non-defective image samples (Golden Sample), and data augmentation technology is used to improve the AI model's recognition of standard samples. The trained AI model can identify the differences between the tested object and the standard sample, locate and mark the position of the micro-crack defect inside the packaged chip, and is completely unaffected by the characteristics of the penetrating image.
Combining smart cameras with deep learning software uses optical character recognition (OCR) to decode damaged barcodes. Thanks to the pre-trained font library of deep learning, the deep learning barcode reading tool in the software is ready to use out of the box, greatly shortening the development time. Users only need to define the target detection area and set the character size. When introducing new characters, you don't need to have visual expertise, and you can also retrain this robust tool to read application-specific barcodes that traditional OCR tools can't decode.
Cognex's Deep Learning OCR tool can use a pre-trained built-in font library of over 1,000 characters to read curved strings, low contrast characters, as well as distorted, skewed, and poorly etched barcodes. The OCR tool also provides a re-training capability, allowing users to solve new or specific characters that cannot be automatically identified on the first pass. Quickly and accurately reading chip identification numbers not only improves traceability, but also ensures the correct information is captured for future reference when needed.
- Real-time autofocus
- Real-time image stabilization function
- Smart measurement function
- Comprehensive observation of bright field and DIC
- Ultra-long depth of field synthesis function
- Ultra-large range puzzle
- Image target navigation
- AI defect target detection
- 3D profile measurement
- Die Bond / Wire Bond multi-layer Chip stacking measurement and inspection can be used, and the AI inspection system can be used to improve the detection accuracy rate to 99.99%
- Distance: arc height, gap between arcs, line spacing, etc., any measurement of plane or step distance
- Abnormality: Wire deviation, ball drop, broken wire and other appearance defects
Traditionally, rule-based machine vision systems used with automated optical inspection (AOI) systems do not perform well. Detecting potential defects (NG) through deep learning can enhance the reliability of the inspection process. The AOI machine uses Cognex Deep Learning tools to identify potential NG situations and provide those images to the system. The defect detection tool can dynamically capture regions of interest, while the classification tool can categorize different types of defects, distinguishing between defective and acceptable wire bonds. Categorizing defects not only helps identify process issues to avoid costly rework downstream, but can also successfully identify defects at the micron level, improving IC chip yield and lifetime performance.
Cognex Deep Learning provides a simple solution that can identify all anomalous features without even having to train on "defective" images. Instead, engineers can use the defect detection tool in an unsupervised mode, training the software using samples of "good" images. Cognex Deep Learning can learn what normal wire bond and lead appearances and positions should be, and flag any deviating features as defective.
Cognex Deep Learning tools can help verify the difference between OK and NG probe marks, making probe mark detection easier and faster. The software is trained on a wide variety of images, including images showing correct probe marks and images showing unacceptable probe marks. Unacceptable marks can then be classified as "pressure related" or "off center".
- The system uses three Allied Vision Guppy F-146B cameras to inspect IC wire bonding.
- The system can count the number of wire bonds, measure their height, and detect any breaks.
Using the Segmentation technology of SolVision AI image platform, the defect features in the image samples are annotated and used to train the AI model. The trained AI model can automatically detect and mark the location of the grain edge fracture defect, which greatly reduces the risk of the chip breaking in the subsequent packaging process.
- Full color/multi-spectral scanning (RGB CCD sensor), wide range of defect detection
- Multiple flash exposure technology, can simultaneously detect defects under different light sources
- Module linearity calibration (CCD), can effectively detect wide range of color difference defects
- Defect stitching is available to inspect large-sized defects
- Integrated encoder, can output defect map
- Unique AI real-time measurement and inspection solution
- Can correspond to “8/12” Wafer/Frame form
- Chip reset position offset/rotation measurement
- Can support Bumping damage/Die chipping detection at the same time
- Detect aperture size according to customer requirements, detection accuracy: 1μm
- Surface appearance defects: foreign objects, opening and closing, bumps, rust, breaks, opening and closing shielding, etc.
Cognex Deep Learning tools provide an easier way to learn and classify chipping and burr marks, as well as distinguish them from normal cutting marks after the cutting process. The software is easy to train and can identify all chipping and burrs, classify them as acceptable or unacceptable, and ignore normal marks within the tolerance range.
The system can detect defects such as slot shape, blockage, thread skew, defects, thread major and minor diameters, pitch, screw head width, thickness, screw bending, and surface scratches. It also provides intelligent inspection data statistics, non-conforming product analysis, report output, and support for remote calibration management.
AI defect classification and judgment solutions can be provided according to the needs of different customers in the production and manufacturing process
Use Solomon SolVision's Segmentation technology to learn about various types of defects, and at the same time set OK categories to avoid false positives and false negatives. With data augmentation, the scope of AI learning is increased. It can not only effectively detect various types of defects, but also accurately detect edge protrusions, black edges or black spots in cluttered or complex backgrounds. It also has a good recognition effect for less obvious defects.
Use the Segmentation technology of the SolVision AI image platform to perform defect identification, detect and mark various subtle defects in complex imaging backgrounds, so that users can monitor and eliminate abnormal conditions of the carrier plate in real time.
The situation of chip jumping in the wafer is random, and the resulting defect patterns are diverse and difficult to predict the location of the defects. For AOI, it is almost impossible to set logic for jump defects and detect them based on it.Using the Segmentation technology of SolVision AI image platform, the AI model is trained with image samples of defects such as stacking, missing materials, skewing and misplacement, and flipping. After the AI training is completed, it can easily and quickly identify and mark the positions of abnormal pick and place on the wafer.
Wafer cutting is a very important process in the semiconductor and optoelectronics industries. If the cutting process cannot maintain high yield, high efficiency and maintain chip characteristics, it will greatly affect the overall production capacity. The quality control of the wafer cutting saw is mainly through the detection of external defects. Common external defects include irregular patterns and multiple drills on the saw body. Since the wafer cutting saw itself has circular stripes, it forms a complex image background, which seriously affects the machine vision for defect detection.Using the Feature Detection tool of SolVision AI image platform, the irregular patterns and multiple drill defects in the image samples are annotated and trained to train the AI model. AI vision can then detect various defects on the wafer cutting saw body in real time.
- Prediction accuracy > 95%
- False positive rate < 5%
- Detect 100 images within 60 seconds (including download, preprocessing, prediction and upload)
Since die bonding technology is the key to the packaging process, it has high requirements for speed and accuracy. However, the texture of the process image is very complex. Traditional optical inspection cannot use logic writing to detect defects such as angle, displacement deviation and missing, which often leads to missed detection, misjudgment and wrong positioning, which greatly affects the production efficiency of the packaging line.Using the Solomon SolVision AI image platform to enhance the reliability of displacement and angle information, accurately detect the manufacturing errors and abnormal conditions of the die bonding system. On the other hand, the AI module can also extend the learning of different chip forms and perform analysis and detection for different types of packaging products.
Combining the AI Image Recognition Platform AIWinOps delivers intelligent manufacturing solutions, applying AI + AOI techniques to industries such as petrochemicals, golf club manufacturing, semiconductors, mechanical part fabrication, and textiles.