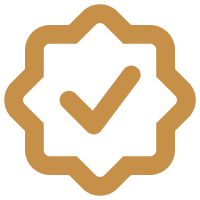
More precise detection of wafer/chip defects
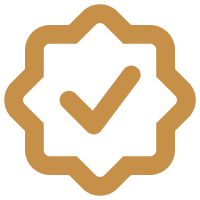
Automated classification of defect types
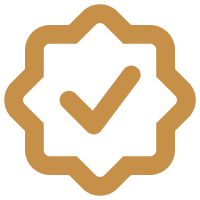
Stronger pattern defect detection capabilities
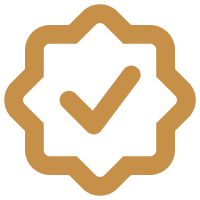
Significantly improved detection efficiency
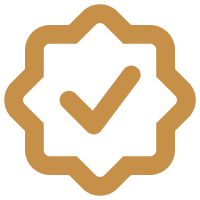
Reduced human intervention and subjective errors
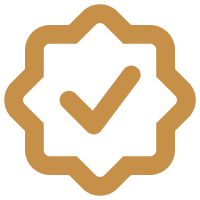
Enhancing defect detection for light guide plates and diffusion boards
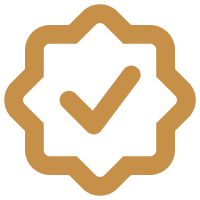
Accurate detection of fine circuit pattern defects
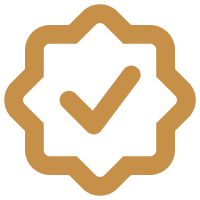
Improved detection rate for color filter and CF defects
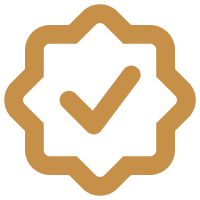
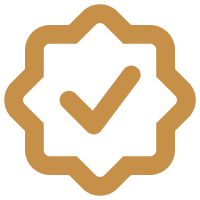
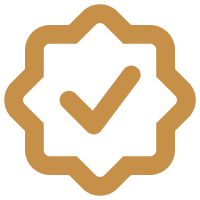
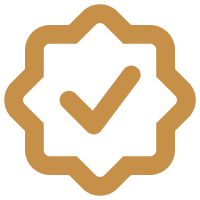
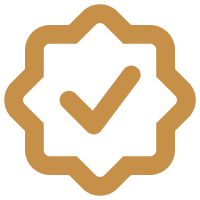
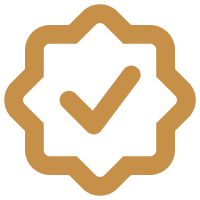
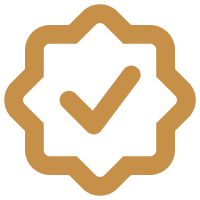
Enhancing defect detection for complex metal/mechanical components
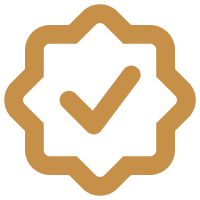
Handling diverse metal materials and surface characteristics
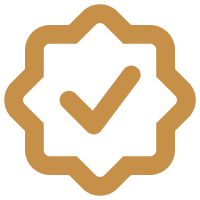
Improving defect detection for critical functional components
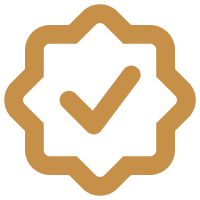
Shortening programming time for complex product inspections
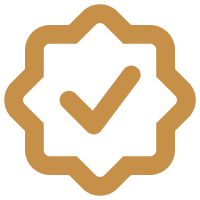
Enhancing high-speed production line inspection adaptability
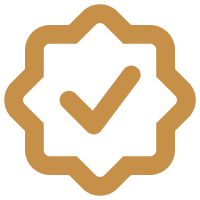
Real-time problem identification and resolution
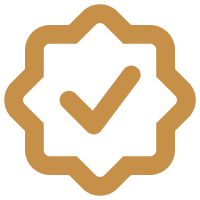
Reducing human errors
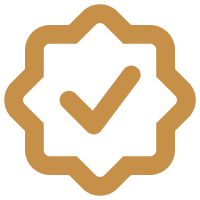
Reducing customer complaints and increasing ROI
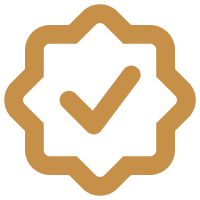
Lowering production costs
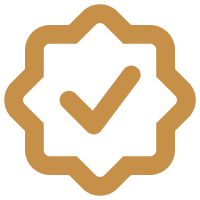
More precise detection of food surface defects
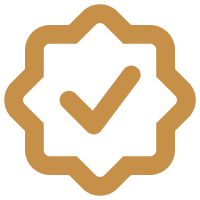
Accurate identification of foreign objects within food
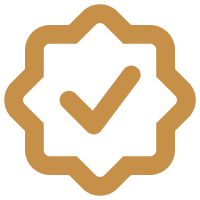
Automated food shape recognition and grading
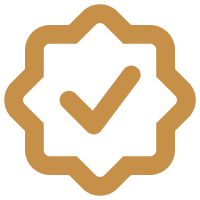
Rapid inspection of food packaging integrity
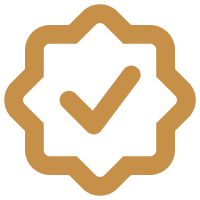
Improved detection of subtle surface defects on medical devices
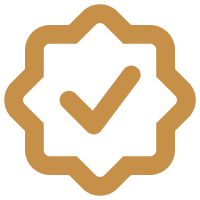
Accurate inspection of medical packaging integrity
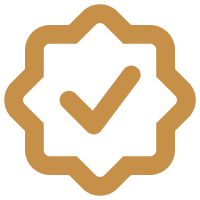
Realization of medical label character recognition
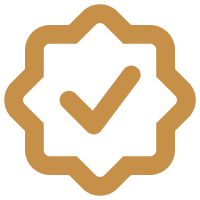
Improved inspection efficiency and reduced human risks
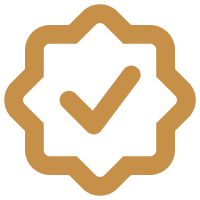
Improved detection accuracy
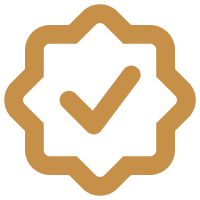
Flexible defect definition and classification
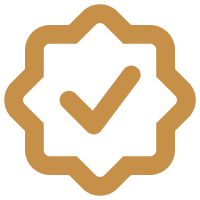
Highly adaptive optimization of inspection
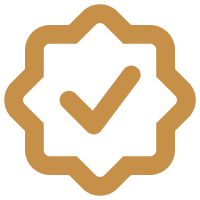
Automated, unattended operation
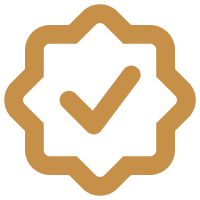
Improved detection of small defects on printed products
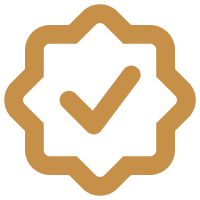
Accurate text/code recognition Labels/printed products often feature text, barcodes, and other encoded information.
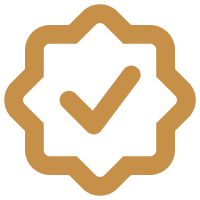
Efficient inspection of complex patterns and image defects
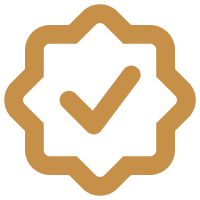
Significantly improved inspection efficiency
Others
Solutions:
The GS2000 can acquire an image of a single wooden board in 0.5 seconds. In image processing and analysis, it uses a neural network classifier and a Bolb module. The neural network is the core of defect detection and classification, and the Bolb can further highlight defects such as holes, cracks, breaks, stains, and gaps, effectively improving the processing efficiency and reliability of the neural network.
The "Digital Twin AI Inspection System" has a set of identical automated systems in both the real world and the metaverse, which operate in real time synchronization. The inspection system uses two vision cameras, one of which is paired with AI inspection software to perform defect detection. The other camera integrates a robotic arm and conveyor belt tracking system to accurately pick up moving PCBs and sort them into OK or NG areas based on the AI inspection results.