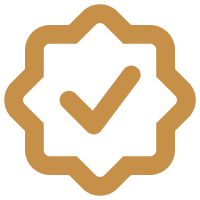
More precise detection of wafer/chip defects
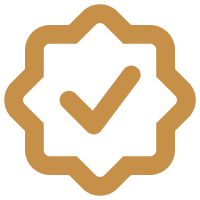
Automated classification of defect types
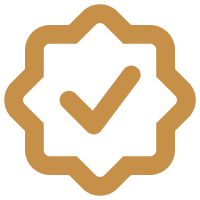
Stronger pattern defect detection capabilities
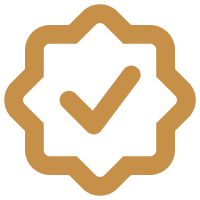
Significantly improved detection efficiency
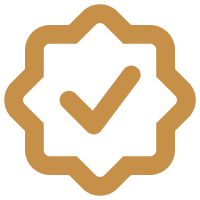
Reduced human intervention and subjective errors
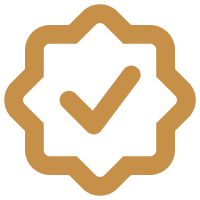
Enhancing defect detection for light guide plates and diffusion boards
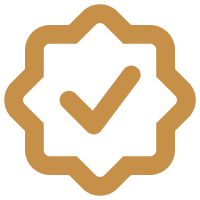
Accurate detection of fine circuit pattern defects
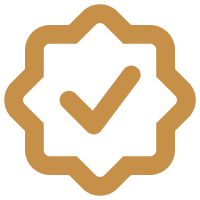
Improved detection rate for color filter and CF defects
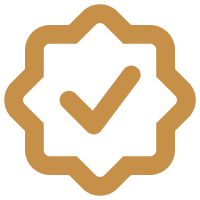
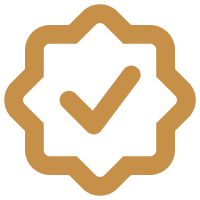
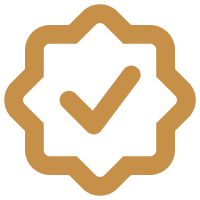
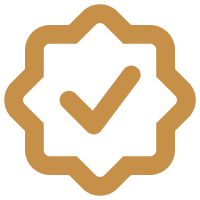
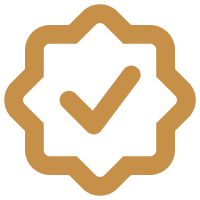
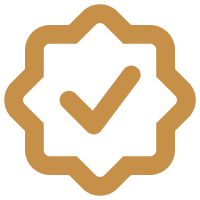
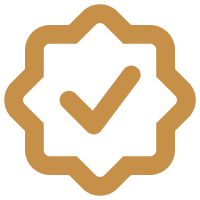
Enhancing defect detection for complex metal/mechanical components
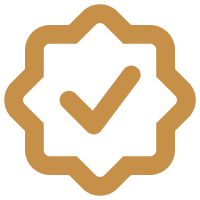
Handling diverse metal materials and surface characteristics
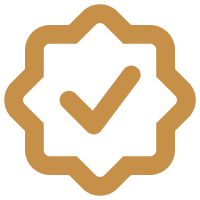
Improving defect detection for critical functional components
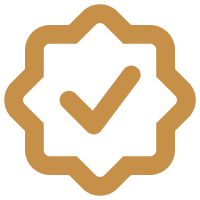
Shortening programming time for complex product inspections
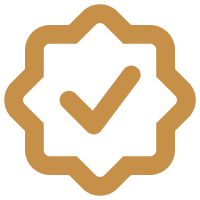
Enhancing high-speed production line inspection adaptability
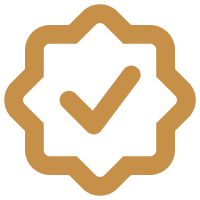
Real-time problem identification and resolution
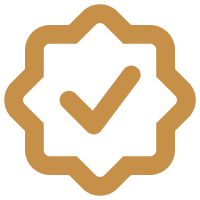
Reducing human errors
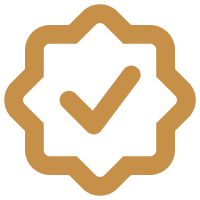
Reducing customer complaints and increasing ROI
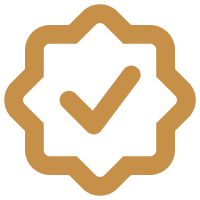
Lowering production costs
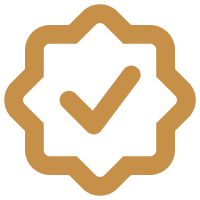
More precise detection of food surface defects
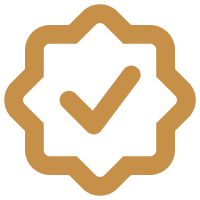
Accurate identification of foreign objects within food
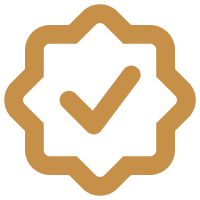
Automated food shape recognition and grading
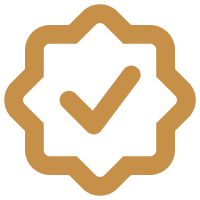
Rapid inspection of food packaging integrity
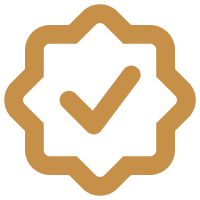
Improved detection of subtle surface defects on medical devices
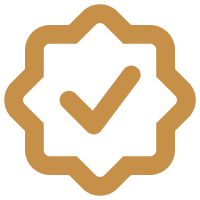
Accurate inspection of medical packaging integrity
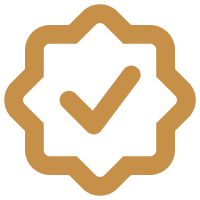
Realization of medical label character recognition
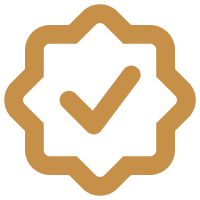
Improved inspection efficiency and reduced human risks
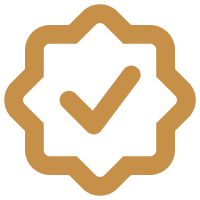
Improved detection accuracy
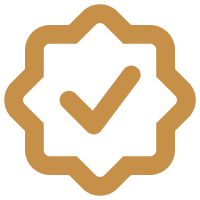
Flexible defect definition and classification
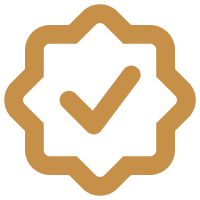
Highly adaptive optimization of inspection
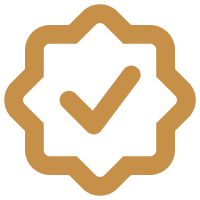
Automated, unattended operation
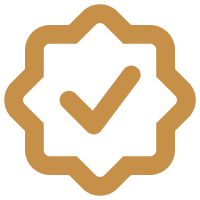
Improved detection of small defects on printed products
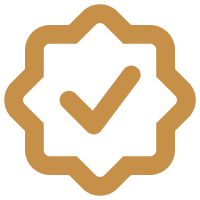
Accurate text/code recognition Labels/printed products often feature text, barcodes, and other encoded information.
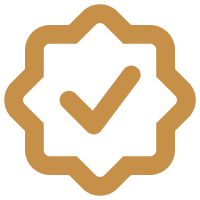
Efficient inspection of complex patterns and image defects
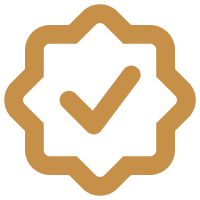
Significantly improved inspection efficiency
Machinery/Vehicles/Metals
Solutions:
Delta AI by RemoteNC is an AI-powered solution that optimizes the management and utilization of engineering drawings. It simplifies the process of finding similar designs, historical pricing, and supplier data, reducing costs and improving efficiency in procurement and R&D.
A new generation of vision systems has been developed that makes machine vision the preferred inspection technology for wheel fastener inspection. The In-Sight 2000 vision sensor can learn the known good appearance of components and compare many different features to determine if they are pass or fail. The In-Sight 2000 is small, has a fully independent light and lens, and is rugged enough to withstand harsh factory environments.
Using Cognex Deep Learning, it is easy to analyze metal piston weld seams by yourself. Engineers can use the Cognex Deep Learning defect detection tool in supervised mode to train the software on a set of "bad" sample images that represent various welding anomalies (including weld overlap) and a set of "good" sample images that do not have any anomalies. In this way, all anomalies, whether they are required or the main cause of rejection, can be identified as defects. In the second part of the inspection, engineers can use the classification tool to classify weld defects by category. By combining the defect detection tool with the classification tool, automotive manufacturers can ensure that the inspection system identifies all welds and successfully classifies overlapping welds.
Due to the high temperature and heat of the welding factory, basic protection must be worn when entering, and the defects of the weld are complex and irregular. It is not easy to maintain the quality consistency by relying on artificial experience to detect the weld.Using SolVision's Segmentation technology, the appearance of normal welds, too thin or too thick welds and no welds are learned to train the AI model. Let the AI learn the characteristics of defects, and quickly detect whether the weld has defects. Pick out the defective welds for repair to effectively control the quality of the welding process.
Cognex In-Sight® vision systems are used for tire assembly inspection, which is typically done by clicking a few times to set up the vision system to inspect the entire tire skin as the roller rotates to combine all the assembly layers. The rugged industrial design of the vision system allows it to perform precise and accurate measurement tasks regardless of the factory production environment.
- High-speed inspection with a maximum inspection capacity of 60PCS/second (2M).
- AI deep learning identifies defects.
- Simultaneous inspection quantity can detect up to 10 areas at the same time.
- Inspection area 2mm2-200mm2.
- The inspection camera uses a 2M-25M high-speed area camera.
- The inspection accuracy camera is equipped with a lens resolution of 0.001mm-0.05mm.
- Measurement capability can detect 2M 60 frames/second or 5M 4 frames/second per second.
- Detailed measurement data classification is stored in different directory folders according to camera sequence, by date and order. Automatically create file names based on date and time.
- NG photo processing automatically generates directory folders and automatically creates file names based on date and time. During the inspection, click on the NG thumbnail or tile in the screen, and the complete photo can be popped up.
- Real-time measurement trend chart can display the data of the last 1000 points of each axis.
- The operation mode has operation mode (only display data), engineering mode (including real-time images) and commissioning mode.
- When imaging, the laser light is projected onto the wheel in a uniform line to ensure that the image has a constant brightness.
- To ensure that the camera receives enough laser light, a high exposure time is required. This can easily lead to stray light from the environment being mixed into the image, but Matrox MIL can effectively remove noise from the image and extract the necessary details.
- Handheld high-precision instrument testing has shown that TreadVIEW can achieve an accuracy of 0.5mm on moving vehicles, with a single image inspection time of approximately 0.5-1 seconds. In terms of specific implementation data, if ±0.5mm measurements can be made within a ±10mm field of view, the system will be able to accurately recommend whether immediate or future remedial action is needed.
Cognex tire solutions use character reading vision tools to enable tire manufacturers to read codes with high accuracy even in the most demanding conditions. The character reading vision tools can accurately locate and read DOT characters, and adapt to the changing code appearance caused by variations in the molding process.
Compact 3D vision solutions must be easy to use and provide software for internal technical personnel to integrate, manage, and maintain. The In-Sight 3D-L4000 works seamlessly with In-Sight software, which is based on a familiar spreadsheet programming methodology. Experienced and novice technicians can quickly and easily set up vision tools. The software then takes over, determining each blob above and below the set plane and highlighting features using a simple user interface (UI) that clearly communicates the health of the production line to every user. True 3D edge and blob tools, as well as other tools designed specifically for 3D imaging that can deliver accurate and consistent results at high speed and high quality, are easy to change and maintain.
Cognex Deep Learning can simplify and automate this identification process. The classification tool can learn to identify and classify different tire tread patterns from a set of training images. It then groups tires with rims according to their category, ensuring that the correct components are selected and installed on the vehicle.
Using industrial cameras and computer vision inspection, the dashboard is measured for height.
SSI Sprocket Series is a continuous image screening machine that is suitable for products that can be hung and have a head diameter greater than 3mm than the rod diameter.
Cognex Deep Learning can quickly and easily detect any anomalies in seatbelt fabric. It is trained on a small set of images of qualified webbing and stitching patterns. The defect detection tool can then immediately detect any errors in the pattern and stitching of the webbing or stitching. If a new webbing or stitching pattern design is introduced, the tool can be trained on a small set of images of the new design and will quickly learn to accept the design, without requiring a long downtime.
- Inspection accuracy with an accuracy of 99.9% or higher.
- OK inspection missed inspection rate is below 0.1%, and NG over kill rate is below 3%.
- The training module takes about 3 months.
- The system has the function of marking the inspection of the entire board and displaying the corresponding OK/NG tile.
A range of cameras can be used to capture images of welds for analysis. While 3D cameras may be required to measure weld volume, 2D cameras are capable of providing all the images required for other defect detection, and can ensure that spot welders are correctly positioned before the process begins.
Allied Vision Manta cameras comply with the GigE Vision communication protocol and support GeniCam functions. They can be cascaded to connect multiple cameras for 360° full inspection without dead angles. This case uses 12 Allied Vision Manta industrial cameras to set up 6 inspection stations to perform defect inspection of screws and fasteners used in automobiles or aerospace. The imaging speed is fast, and up to 500 can be detected per minute.
Semi-automatic inspection equipment, fully automatic discharge, high-speed and high-precision detection, precise defect marking, authority management, size confirmation, appearance inspection, intelligent inspection data statistics, non-conforming product analysis, report output, support for remote calibration management
SCI series is a continuous screw cap optical image screening machine
- High-speed inspection with an inspection capacity of 30 meters/minute.
- Maximum inspection width 360mm.
- Maximum roll diameter 450mm.
- High-resolution line scan camera uses color 8K and black and white 16K.
- Learning function has AI artificial intelligence function to learn to identify OK/NG products, and then inspect defects.
- Inspection capability can detect defects of 0.12mm2 for color and 0.06mm2 for black and white.
- Defect threshold can set the defect length and width threshold, and all above the threshold can be detected.
- Defect marking has defect marking capability, and you can choose to use a brush or laser marking.
- Management function has management level, engineer and operator level, and can set operation permissions.
- Real-time display has real-time display of scan image thumbnails and full images.
- Automatic recording can record the number of defects, and the statistics table and curve chart are displayed on the screen.
- Defect photos can be selected whether to save the defect photos.
- High-speed inspection with a maximum inspection capacity of 1000PCS/minute.
- Inspection turntable diameter 250mm-700mm.
- Standard machine size 850mm×width 850mm×height 1800mm.
- High-resolution area camera uses 1.3M-25M.
- Learning function has AI automatic learning function to identify OK products and then check NG defective products.
- Inspection capability can detect defects of 0.01mm2 for color and 0.005mm2 for black and white.
- Defect threshold can set the defect length and width threshold, and all above the threshold can be detected.
- Defect marking has defect marking capability, and the selector can be used to separate the materials into OK/NG/NULL three hoppers.
- Management function has management level, engineer and operator level, and can set operation permissions.
- Real-time display has real-time display of inspection thumbnails and full images.
- Automatic recording can record the number of defects, and the statistics table and curve chart are displayed on the screen.
- Defect photos can be selected whether to save the defect photos.
Tires go through many high-pressure, high-load and high-temperature processes during the manufacturing process. The dust generated by the on-site machines and materials, coupled with the long-term operation of the printing process, make the surface of the inner tube blurry and the color shades uneven, affecting the recognition of the inner tube coding. After mass production, it is not conducive to manual recognition. If traditional AOI detection is used, it cannot be effectively identified in the case of unclear digital printing.Using Solomon SolVision's Segmentation technology, the numbers and shapes of the tire inner tube codes are photographed and trained for AI models. Then, optical character recognition (OCR) is used to accurately identify the codes. Even for incompletely printed or lightly colored characters, they can all be successfully identified, effectively improving the accuracy of code recognition.
Engine numbers are printed on the engine by branding. When taking images of engine numbers, they are also easily affected by shadows, resulting in uneven brightness of fonts and backgrounds, which makes it impossible to read the numbers by machine. Even with artificial visual inspection, it is still difficult to quickly identify the codes on the engine on the production line.Using the Segmentation technology of Solomon SolVision AI image platform, the model is trained with image samples of different brightness and optical character recognition (OCR) is performed to convert the engine number in the image into numerical information, which is immediately logged into the original database system and linked to the VIN.
The system is able to first take pictures of the parts, and then create a model (Golden sample) based on them. Then, it can use this as a reference to identify and align the same parts, and at the same time perform dimensional measurements. The parts that are inspected and measured can be used for assembly; the abnormal ones are first sent back to the parts storage area.
Cognex Deep Learning can learn from a set of images of installed spring clamp pliers that are qualified and unqualified. The classification tool can quickly determine whether the clamp is working properly or identify potential problems that need to be corrected before further assembly of the vehicle can proceed.
Cognex Deep Learning provides an effective inspection solution that combines the human ability to identify subtle variations with the reliability, consistency, and speed of an automated system. Engineers can use the Cognex Deep Learning software's defect detection tool in supervised mode to train the deep learning-based software on a set of representative "good" and "bad" compression ring images. Technicians can add annotations to "bad" images where there are long scratches, and to "good" images with normal variations and allowable defects such as rust and small cracks. Based on these images, Cognex Deep Learning can learn the natural shape and surface characteristics of pistons, as well as the typical appearance of scratches.
High-precision PIN inspection machine designed specifically for PINs, fine inspection, to ensure product quality
Cognex Deep Learning's text and character reading capabilities can reliably and accurately decode deformed, skewed, damaged, or low-contrast codes. This can be done by training on a set of OCR code images with different angles, light sources, damage conditions, and other variations.
NGI Glass Disc Series is a series of glass disc inspection machines that use the light transmission properties of glass to automatically detect defects on both the top and bottom of the glass disc.
- Image recognition rate > 95%
- Model adjustment
- Intelligent classification
- Can detect pores, holes, notches, cracks, micro-cracks, material surface indentation, dirt, scratches, foreign body adhesion, material deformation
NCI Conveyor Belt Series is an entry-level automatic inspection machine that is ideal for budget-conscious buyers who need to inspect products for single-sided defects.
Metal case scratches are very fine. Under normal light, it is difficult for personnel to detect defects visually because the metal material is easy to reflect light, which can easily lead to poor appearance quality problems.By using SolVision's Segmentation technology, a defect defect database is established for the appearance shape of defects, and specific defects are classified, such as obvious defects, fine defects and extremely fine defects. Deep learning is used to identify obvious defects and ignore acceptable minor defects. , Effectively improve the detection accuracy and speed, and ensure that the finished products on the production line can enter the assembly process without any defects.
Metal stamping parts can have a wide variety of defects in different shapes and sizes, and oil and water stains are even more difficult to observe. On the other hand, the brightness of the workpiece also varies during imaging, which makes it very difficult to perform defect detection.By using the Segmentation technology of SolVision AI image platform, AI models are trained with images of various defects with different brightness. After training, the AI model can easily detect various defects on stamping parts, such as: shallow scratches, oil stains, water stains, burrs, etc., which greatly improves the surface quality of the product.
Matrox DA software enables real-time positioning on the production line, allowing for a variety of related inspection applications, including appearance and dimensional measurement, hole detection, hole size inspection, and product counting.
By using the Segmentation technology of SolVision AI image platform, the fine defects on the club head in the image samples are labeled one by one, and the AI model is trained. After the training is completed, the AI model can locate and mark all the fine surface defects regardless of the brand logo, design pattern and metal gloss.
- Matrox Imaging's software, hardware components and deep learning systems use deep learning technology to expand the field of image processing. Through systematic construction, it greatly saves cost, time and manpower, and at the same time greatly improves product quality.
- By collecting more than 8,000 images of parts, manually marking and classifying them as OK and NG, the team used the interactive environment of MIL CoPilot to train and build models, and finally imported the models into Matrox DAX for automatic classification of new images.
Cognex Deep Learning is trained on a series of different images with different angles and rotation directions, and the classification tool can reliably classify painted colors. Then, when making a selection, Cognex Deep Learning will examine the entire image and appropriately weight the various changes, reflections, refractions, granularity and chromaticity within the image to select the best match.
VisionPro Deep Learning is highly customizable software that uses artificial intelligence (AI) to analyze thousands of annotated images to detect defects in challenging environments, such as the surfaces of cylindrical and prismatic battery cells. EtherInspect is a VisionPro-enabled vision software that uses built-in templates and tools to accelerate deployment. When used with modular 2D hardware like the In-Sight D900, these solutions allow users to quickly process EoL electric vehicle battery inspection. When robust defect detection is more important than speed, 3D solutions like the In-Sight 3D-L4000 can provide more accurate and precise measurements and surface inspection.
The short exposure time of Cognex line scan industrial cameras (CICs) makes them ideal for high-speed continuous operations such as electrode coating inspection. Combined with VisionPro deep learning software, the solution can isolate subtle defects in poor contrast environments, such as matte black electrode coatings.
Cognex Deep Learning uses color cameras to accurately verify that winding processes have been completed flawlessly. The defect detection tool can learn from a set of training images that includes images of zero-defect winding, as well as images that are labeled for a variety of other possible defects that can appear in different locations, including overlaps, misalignments, and crossovers.
ECS Eddy Current Sorting Machine is used to detect heat treatment quality, hardness, and material mix-up.
Cognex Deep Learning can quickly identify pores under the same lighting conditions, while other methods are still trying to detect them. Engineers can use a set of "good" and "bad" cylinder images to train the software in a few minutes, using the mask filter program to adjust the region of interest and eliminate the bright circular plane with a hollow center. Technicians can use the Cognex Deep Learning defect detection tool in supervised mode to annotate the pores in the "bad" images and adjust the parameters, including feature size, aspect ratio, aspect ratio and shear modulus, to ensure that the created reference model takes into account all appearance changes. "Good" images that describe normal cylinders can help the software learn what kind of minor casting anomalies and variations are acceptable. Engineers can retrain the system, adjust parameters and add additional images until the model can summarize the normal appearance of the cylinder and identify abnormal conditions. At runtime, the deep learning-based software can detect each image in milliseconds, depicting features with pores as defects and other features as normal.
- High-speed inspection with a maximum inspection capacity of 1PCS/second (2M)
- AI deep learning identifies defects.
- Adjustable inspection track.
- Inspection area 2mm2 -200mm2.
- The inspection camera uses a 2M-25M high-speed area camera.
- The inspection accuracy camera is equipped with a lens resolution of 0.001mm-0.05mm.
- Measurement capability can detect 2M 60 frames/second or 5M 4 frames/second per second.
- Detailed measurement data classification is stored in different directory folders according to camera sequence, by date and order. Automatically create file names based on date and time.
- NG photo processing automatically generates directory folders and automatically creates file names based on date and time. During the inspection, click on the NG thumbnail or tile in the screen, and the complete photo can be popped up.
- Real-time measurement trend chart can display the data of the last 1000 points of each axis.
- The operation mode has operation mode (only display data), engineering mode (including real-time images) and commissioning mode.
There are many kinds of defects in the manufacturing process of hard disk brackets, including metal indentation, surface white fog, hole scaling, hole blackening, etc. It is not easy to detect them one by one through manual inspection. However, small defects may cause problems such as misalignment of pores during assembly.Using SolVision's Segmentation technology, the AI model is trained for the shape of defects on the metal bracket. After the AI learns the defect features, it can quickly detect various small defects on the hard disk metal bracket.
- Semi-automatic manual feeding
- Size confirmation, color identification
- In-line conveyor belt inspection
- Easy to install, low maintenance cost
- Appearance inspection, defect detection
At present, manual identification and registration of bicycle frame numbers are still used, which is labor-intensive and inefficient. If automatic optical identification (AOI) is used for character recognition, the stainless steel tube surface is a curved surface, and lighting is easy to cause reflection, making the The code on the curved surface is not clear. Whether manual or automatic optical inspection, it is more difficult to identify the characters on the curved, reflective stainless steel tube. Solomon combines machine vision and artificial intelligence to use SolVision Segmentation technology to train AI models for the gloss of the numbers on the stainless steel tube, which can achieve excellent optical character recognition results.
Cognex In-Sight series stand-alone 2D machine vision systems are embedded with advanced software like BeadInspect to quickly analyze bead shape, size, and appearance. For more robust inspection, many 2D systems can be equipped with VisionPro deep learning, an image analysis software that uses artificial intelligence (AI) to separate defects from acceptable beads by comparing thousands of "good" and "bad" images. When the inspection system needs to measure the height or profile of beads, 3D machine vision systems like the In-Sight 3D-L4000 are the most effective solution, using a blue laser without spots to capture high-quality images and minimize glare.
Fully automatic inspection equipment, fully automatic loading and unloading, high-speed and high-precision inspection, precise defect marking, real-time yield statistics chart, authority management, size confirmation, appearance inspection, intelligent inspection data statistics, defect analysis, report output, support remote calibration management
The roof of a car is a streamlined shape, and its weld has a high and low drop, so the brightness of the images taken at each position is different. The randomly generated air hole defects also have different characteristics, so it is difficult to formulate rules for detecting weld air hole defects in this situation.Using the Segmentation technology of Solomon SolVision AI image platform, the weld air hole defect images of various brightness are annotated to train the AI model, which can detect air hole defects anywhere on the weld regardless of the image brightness.
Cognex Deep Learning can reliably verify the correctness of assembled wheel bolts. The component location tool can be trained using example images of bolts in different positions and angles. Once completed, it can reliably identify the presence or absence of each wheel bolt in a complex and difficult-to-distinguish field of view, and confirm that the number of bolts is correct and that they are located in the correct position on the wheel.
Laser welding is divided into two categories: laser heat conduction welding and laser deep penetration welding according to the power density. It has different weld seam characteristics. Due to the different welding positions and styles of the products, it is impossible to use traditional optical detection to distinguish the annular distribution of the weld seam, the appearance of the finished product such as no welding, etc., so the production line is finally inspected manually, which often causes the welding quality to be uneven.By using the Classification and Segmentation technology of Solomon SolVision AI image platform, AI models can be trained with weld feature images to identify welding power and no welding defects, and the number and distribution of fish scale patterns of weld seams can be accurately detected through deep learning.
- Cognex Deep Learning can be trained on the full range of possible engine types and component configurations. The distance between the components and the center distance can cause the wide-field lens to present the engine components at different angles. No matter what angle the components are presented at, the classification tool can learn to identify each component.
- In order to accurately perform engine assembly verification, the classification tool must learn the engine type and the required locations of different oil filters, wires, hoses and other components, and immediately mark any engines with missing or incorrectly installed components. This allows errors to be identified before the engines are ready to be installed in vehicles.
- Measure various features of turbine blades
- Inspect blades for defects and flaws
- Verify that the characters on the parts are correct
The side threaded surface of metal threaded kits is easily damaged by collision during handling, or by the tool marks of the cutting tool during processing. Due to the fact that cylindrical kits are easy to roll and are small and difficult to hold, even with strong light and microscope equipment, it is still difficult to detect with the naked eye, and it is easy to miss and miss detection.Using Solomon SolVision, AI models are trained with images of metal kit defects, and Segmentation technology is used to learn the characteristics of tool marks and collision defects. After the AI model is trained, it can easily detect small metal defects that are difficult to identify with the naked eye. The kits are picked out to improve the overall quality of the shipped metal kits.
- Prediction accuracy > 95%
- False positive rate < 5%
- Detect 100 images within 60 seconds (including download, preprocessing, prediction and upload)
Combining the AI Image Recognition Platform AIWinOps delivers intelligent manufacturing solutions, applying AI + AOI techniques to industries such as petrochemicals, golf club manufacturing, semiconductors, mechanical part fabrication, and textiles.
- Developing and implementing AOI machines used for research and incoming inspection purposes
- Capable of detecting wear and tear, partial damage, fractures, or foreign materials on spring probes
- Spring probe diameter (tip): >=0.1mm, compatible with multiple crown-shaped heads
- Detection coverage and velocity: X axis: minimum 10 spring probes; Y axis: 2.3 millimeters/second; Z axis: manual adjustment